Non-conscious cognition is becoming an increasingly common interface between humans and the world's many archives, formats and repositories; architects should not miss out on this opportunity for collaborative intelligence, intelligence whose strengths span beyond just its processing powers. The first step toward a successful machine-human collaboration in architectural heritage studies, where architects work alongside AI rather than use it as a tool, is understanding synthetic cognition's agency in data analysis. With the emergence of machine vision systems, architects have now arrived at an opportunity where historical repositories of architectural heritage can be carefully inspected by the eye of the new cognitive agent, the algorithm.1 Through methods of precedent analysis, architectural history relies upon the categorisation of its attributes such as style, use or programme informing future design. In the times when perception is no longer an anthropocentric domain, should the architects not be engaging non-human agents to extend this practice, challenge and unlearn biases in the reading of architectural history?
In the times when perception is no longer an anthropocentric domain, should the architects not be engaging non-human agents to extend this practice, challenge and unlearn biases in the reading of architectural history?
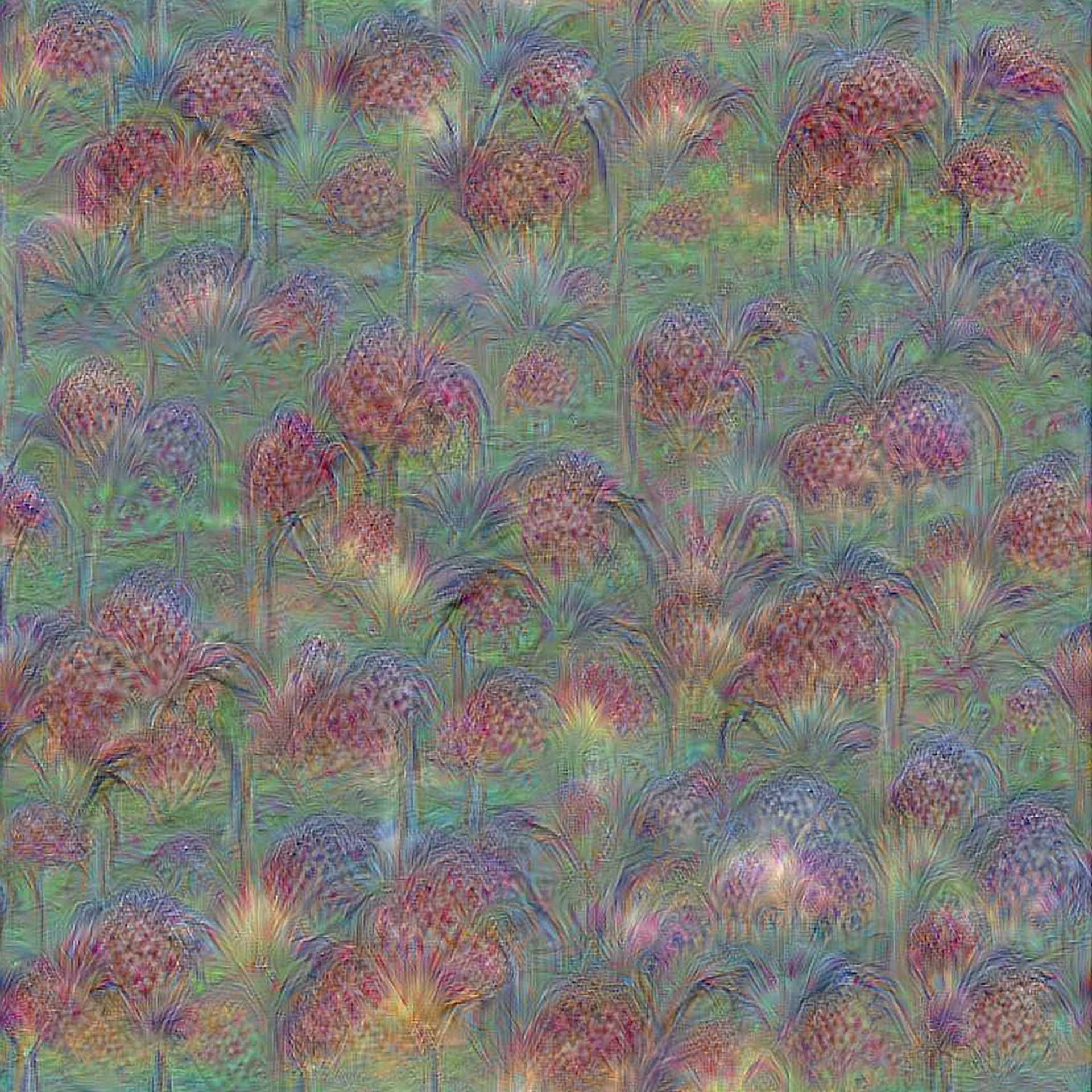
Fig. 1. Gothic Architecture
In his book Projective Cast (1995) Robin Evans recalls Sigfried Giedion's belief that each epoch was characterised by an "irresistable force", an encoded paradigm shift that transcends the work of all disciplines.2 While Giedion points out that the glazed corners of modernist architecture, cubism and relativistic physics were all characterised by "planarity, transparency, and simultaneity", Evans notes that the embodied paradigm was only recognised from a perspective of the next era, decades after the works were completed.3 If the properties of architectural forms can continue to be discovered and reinterpreted without the geometry itself changing, is precedent analysis not just a reiterative process of "pattern recognition" and "anomaly detection"4 of the cultural traces embodied within the architectural forms?
To define the limits and boundaries within which artificial cognition operates, it is essential to understand the flows of the anthropocentric model of thought.
To define the limits and boundaries within which artificial cognition operates, it is essential to understand the flows of the anthropocentric model of thought. In his pivotal book Style and Epoch (1924), Moisei Ginsburg points out that the historical style penetrates contemporary architecture, not only through its ethos but as an everyday experience that the architect cannot escape. Even though modern architecture lies beyond the classical Greco-Italian system, traces of European heritage live within the very fabric of it, and as such, within any “mortal” that experiences them. In a similar vein, Erwin Panofsky deemed art historians' susceptibility to bias as the reality in which they are immersed cannot be escaped, nor can the lived experience of it and it takes training for an expert to unlearn their preconceptions.5 The artificial gaze, free of any prejudices, offers precisely what Panofsky has sought.
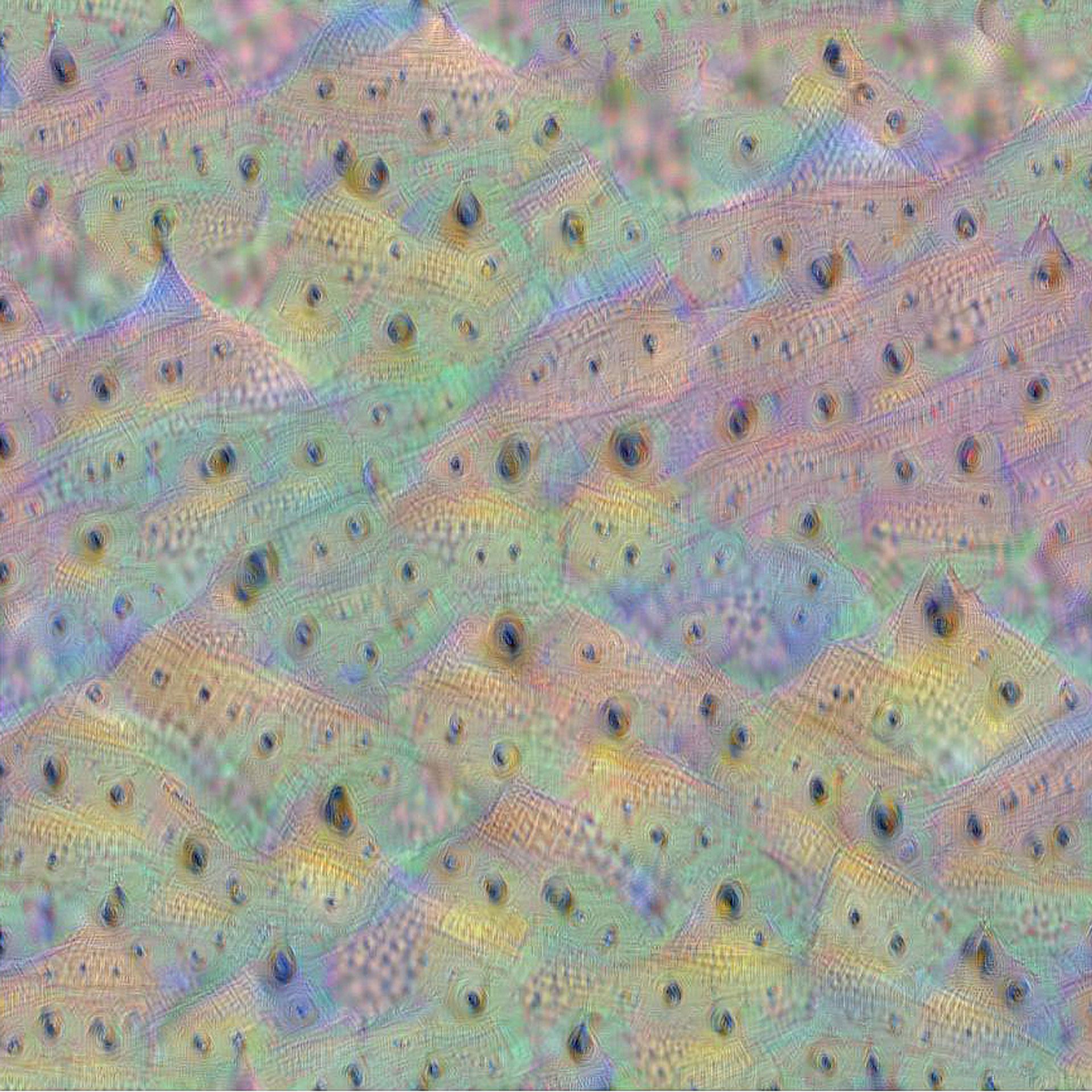
Fig. 2. Renaissance Architecture
Machine vision systems interact with visual information in a manner profoundly different to humans. They are trained on sets of examples from which they deduce and synthesise patterns of relations to build their understanding of the subject. Algorithms learn with a certain level of autonomy, but they will only ever know what is defined within the given dataset, nothing outside of it. The most valuable contribution to the cultural analysis of architectural heritage stems precisely from this alternative perceptual model. A trained algorithm analyses and classifies images by feeding them through numerous layers of its structure; correlating patterns with activations of the training phase, the algorithm decides on the probability of the class to which the image belongs to. Those activations can be visualised as an abstract map of what, at this point, is no longer an image but an operation of two functions, a mathematical equivalent of the act of looking.
To investigate this act of looking in the context of architectural heritage, a machine vision algorithm was trained to differentiate between four architectural styles; gothic, renaissance, baroque and modernism.
To investigate this act of looking in the context of architectural heritage, a machine vision algorithm was trained to differentiate between four architectural styles; gothic, renaissance, baroque and modernism. To explore its artificial gaze, the image recognition process was reversed through the method referred to as DeepDream.6 An algorithm was prompted to plot images that encapsulate all of the activations for each style, a visual representation of the patterns it found and deemed relevant during the learning phase.
The first notable characteristic of all returned images was their lack of depth. The four styles were represented as a composition of fractal-like patterns with a clear correlation to each style's aesthetic. None of them showed actual buildings. The algorithm had no preconception of the rules of perspective, and it could only comprehend the contents of an image for what it was; a two-dimensional map of pixels.
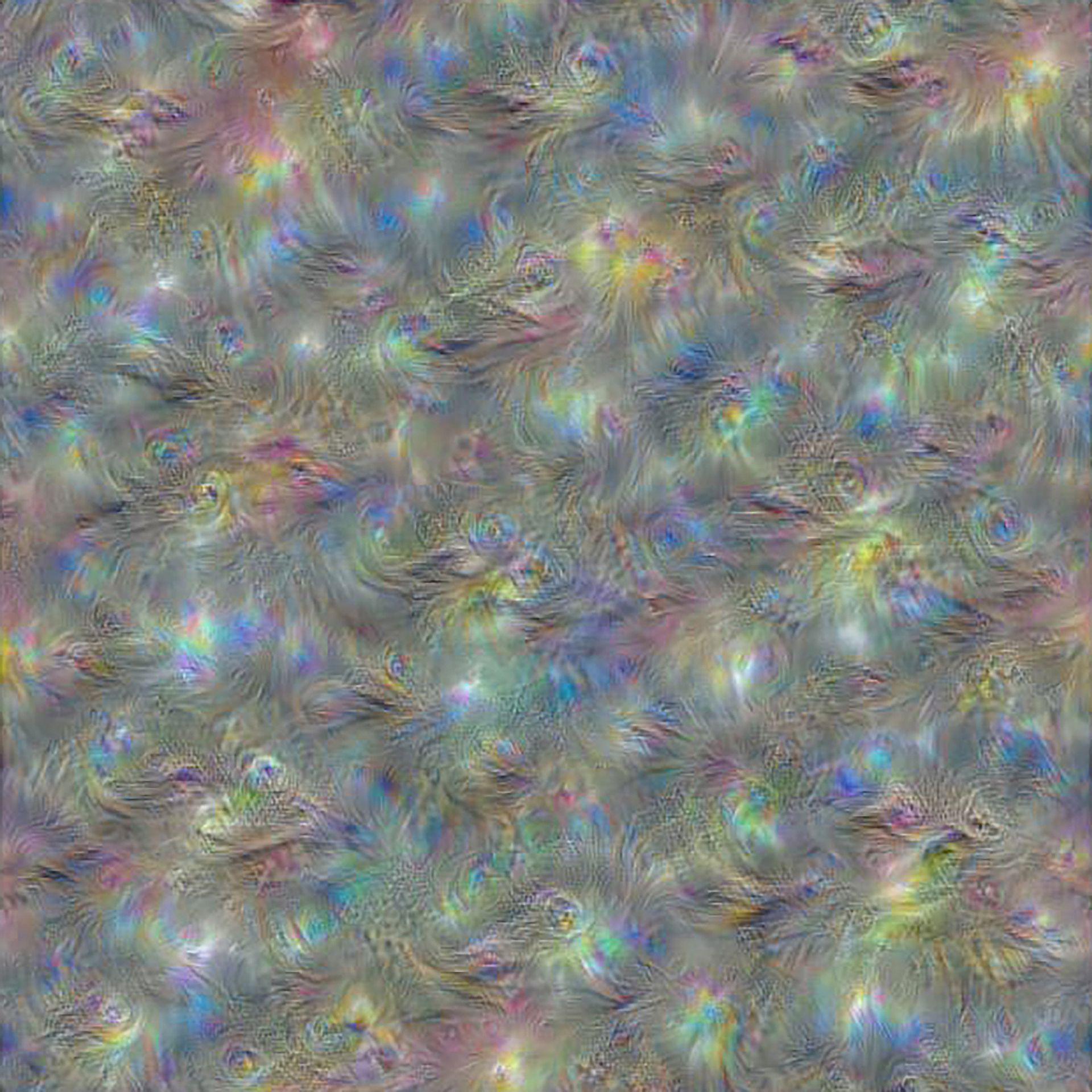
Fig. 3. Baroque Architecture
A reserved approach to ornament characterises renaissance and modernist buildings, and in both cases, the algorithm picked on the buildings' overall geometry as those are often captured from afar, showing the composition of their massing.
The second pattern picked up by the algorithm was the correlation between the style's aesthetics and the photographic conventions used to capture them. Less ornate styles are known to be appreciated for the proportions and composition of their constituting planes, and styles famous for their flamboyant ornaments are documented in much more detail using techniques such as close-ups. A reserved approach to ornament characterises renaissance and modernist buildings, and in both cases, the algorithm picked on the buildings' overall geometry as those are often captured from afar, showing the composition of their massing. In the case of baroque and gothic the activations were arranged into flamboyant patterns resembling rose windows and ornaments, all usually found at a smaller scale. This study reiterated some already well-formulated findings in semiotic studies, but more importantly, it highlighted that the agency of artificial cognition stems from its perceptual process rather than its processing power.
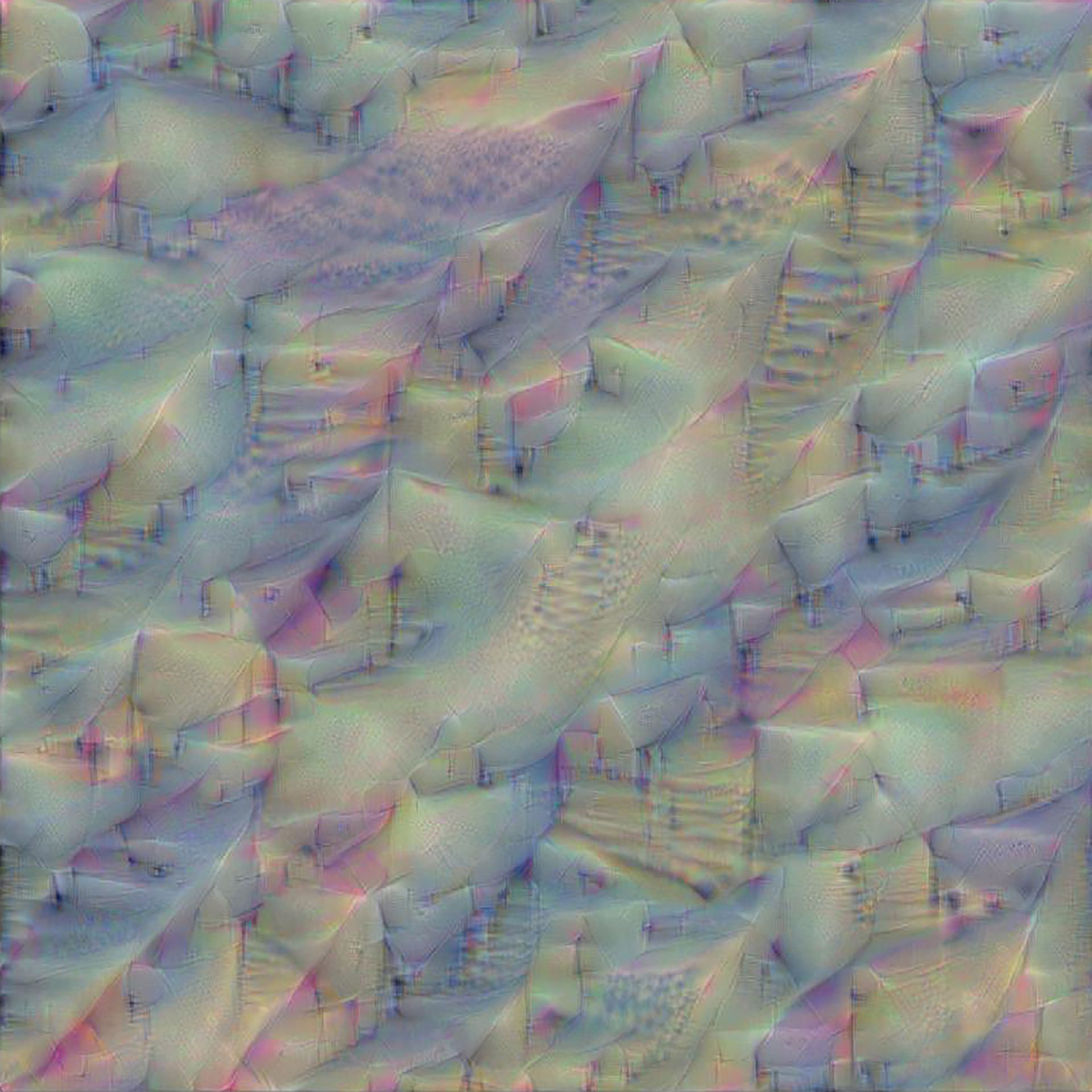
Fig. 4. Modernist Architecture
Non-human pattern detection allows architectes to not only expand on the existing knowledge but, through the alternative cognitive processes, “unlearn” the biases by making them visible.
Either through reference or repudiation, architectural practice sits within the context of its past. Perceptual experience plays a critical role in the visual assessment of culture, and human experiences are vastly influenced by their previous associations affecting how the analysed subject is assessed. Non-human pattern detection allows architectes to not only expand on the existing knowledge but, through the alternative cognitive processes, “unlearn” the biases by making them visible.
Read the entire "Architecture of Machine Dreams" column by Gosia Starzyńska.
Notes
1 Matteo Pasquinell. “The Eye of the Algorithm: Cognitive Anthropocene and the Making of the World Brain.” November 5, 2014. matteopasquinelli.com/eye-of-the-algorithm/.
2 Robin Evans, The Projective Cast: Architecture and Its Three Geometries (Cambridge, MA, USA: MIT Press, 1995), 57.
3 Evans, The Projective Cast: Architecture and Its Three Geometries, 59.
4 Matteo Pasquinell."Anomaly Detection: The Mathematization of the Abnormal in the Metadata Society." shorturl.at/uzDKP
5 In her 2017 paper Dream Formulations and Deep Neural Networks: Humanistic Themes in the Iconology of the Machine-Learned Image Emily L. Spratt points out that the pre-existing biases of human perception in the classification of art history dentified by Erwin Panofsky in his book Iconography and Iconology: An Introduction to The Study of Renaissance Art (1955) can also be made visible through the machine vision studies of art.
6 In 2015 Google’s AI division DeepMind developed a method to visualise the learning process of an image recognition algorithm. The program, referred to as DeepDream, reversed the machine vision system which allowed it to generate rather than classify images. The project aimed to answer what the algorithm was “seeing” in an examined image.
Bibliography
Crary, Jonathan. Suspensions of Perception: Attention, Spectacle, and Modern Culture. October Books. Cambridge, MA, USA: MIT Press, 1999.
Evans, Robin. The Projective Cast: Architecture and Its Three Geometries. Cambridge, MA, USA: MIT Press, 1995.
Ginzburg, Moisei. Style and Epoch. Translated by Anatole Senkevitch. Oppositions Books. Cambridge, MA, USA: MIT Press, 1983.
Panofsky, Erwin. Studies In Iconology: Humanistic Themes In The Art Of The Renaissance. 1st edition. New York: Westview Press, 1972.
Pasquinelli, Matteo. “The Eye of the Algorithm: Cognitive Anthropocene and the Making of the World Brain.” November5, 2014. matteopasquinelli.com/eye-of-the-algorithm/.
Spratt, Emily L. “Dream Formulations and Deep Neural Networks: Humanistic Themes in the Iconology of the Machine-Learned Image”, Kunsttexte - Renaissance, (2017). https://doi.org/10.18452/18693.
List of figures
Fig. 1. Gothic Architecture
DeepDream technique applied to dataset set containing images scraped from online repositories for words Gothic Architecture. ©Gosia Starzyńska, 2020.
Fig. 2. Renaissance Architecture
DeepDream technique applied to dataset set containing images scraped from online repositories for words Renaissance Architecture. ©Gosia Starzyńska, 2020.
Fig. 3. Baroque Architecture
DeepDream technique applied to dataset set containing images scraped from online repositories for words Baroque Architecture. ©Gosia Starzyńska, 2020.
Fig. 4. Modernist Architecture
DeepDream technique applied to dataset set containing images scraped from online repositories for words Modernist Architecture. ©Gosia Starzyńska, 2020.
Bio
Gosia Starzyńska is an architect, an educator and a current researcher at the Laboratory for Design & Machine Learning at the Royal College of Art in London. She is also an associate lecturer at Oxford Brookes University where she leads machine learning and data-driven design teaching curriculum for the Architecture Master’s degree apprenticeship course. In her research work and teaching practice, Gosia explores the transdisciplinary advancement in AI and applications of machine learning in architectural theory and practice.