Learning architecture has always been a multi-faceted scholarship. Over two thousand years ago, in one of the most famous books on architecture, De Architectura, Vitruvius outlined the critical components of architects’ training. He was to be versed in both theory and practice of his craft, but above all, well educated in various disciplines. "Let him be educated, skilful with the pencil, instructed in geometry, know much history, have followed the philosophers with attention, understand music, have some knowledge of medicine, know the opinions of the jurists, and be acquainted with astronomy and the theory of the heavens."1 Architecture continued to honour those principles, and as new disciplines emerged, they would make their way into the constantly expanding curriculum. Today, as novel technologies of AI are shifting paradigms in all aspects of contemporary life, architects must develop methods to address this within architectural education.
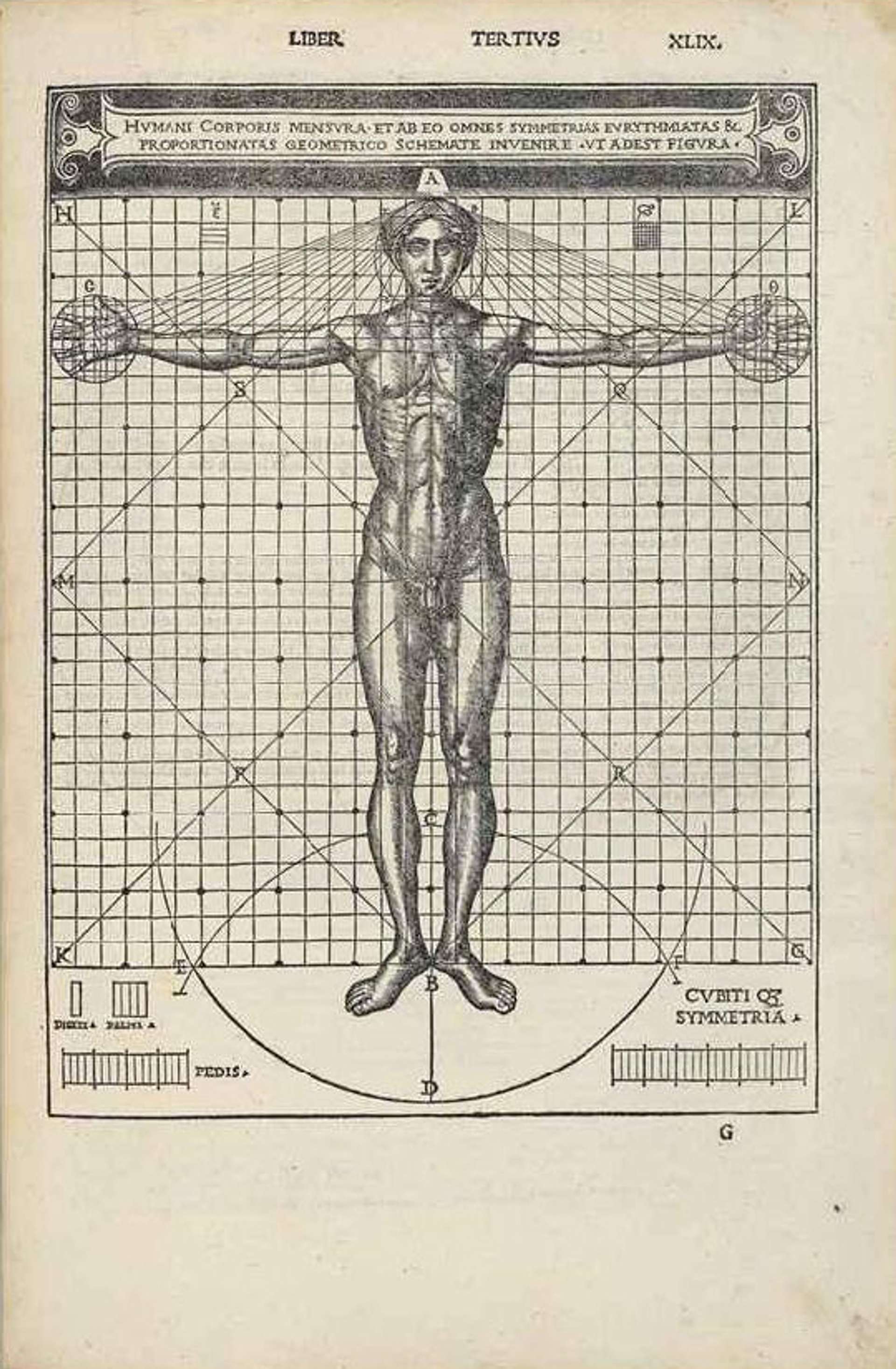
Fig. 1
Contemporary architectural education still follows the Vitruvian path, embracing practice alongside technology, engineering, philosophy and art.
Contemporary architectural education still follows the Vitruvian path, embracing practice alongside technology, engineering, philosophy and art. "Good architectural education should have the exploration and indulgence of the art school, the technical and pragmatic considerations of the science lab, the lawyer's chambers and the builder's hut," writes Neil Spiller in his introduction to the collection of essays Educating Architects (2014). As technology and science expand, new aspects come to the forefront. Contemporary philosophy branched out into metadata society2 and philosophy of AI; medicine grew into neurobiology, working hand in hand with cognitive sciences. Architectural practice is already populated with novel drafting and calculating tools, each affording new agencies. There are two ways in which novel AI systems can be integrated within architectural education; on the one hand, architects should learn about the machine and its workings, and on the other, they should understand their role in educating algorithms. As architects continue with the Vitruvian approach and expand their knowledge, they should also consider taking under their wings the new generation of emerging learners; algorithms.
Unlike the hard-coded models of the 90s, contemporary AI is much less dependent on rule-based programming and much more self-reliant in processing data.
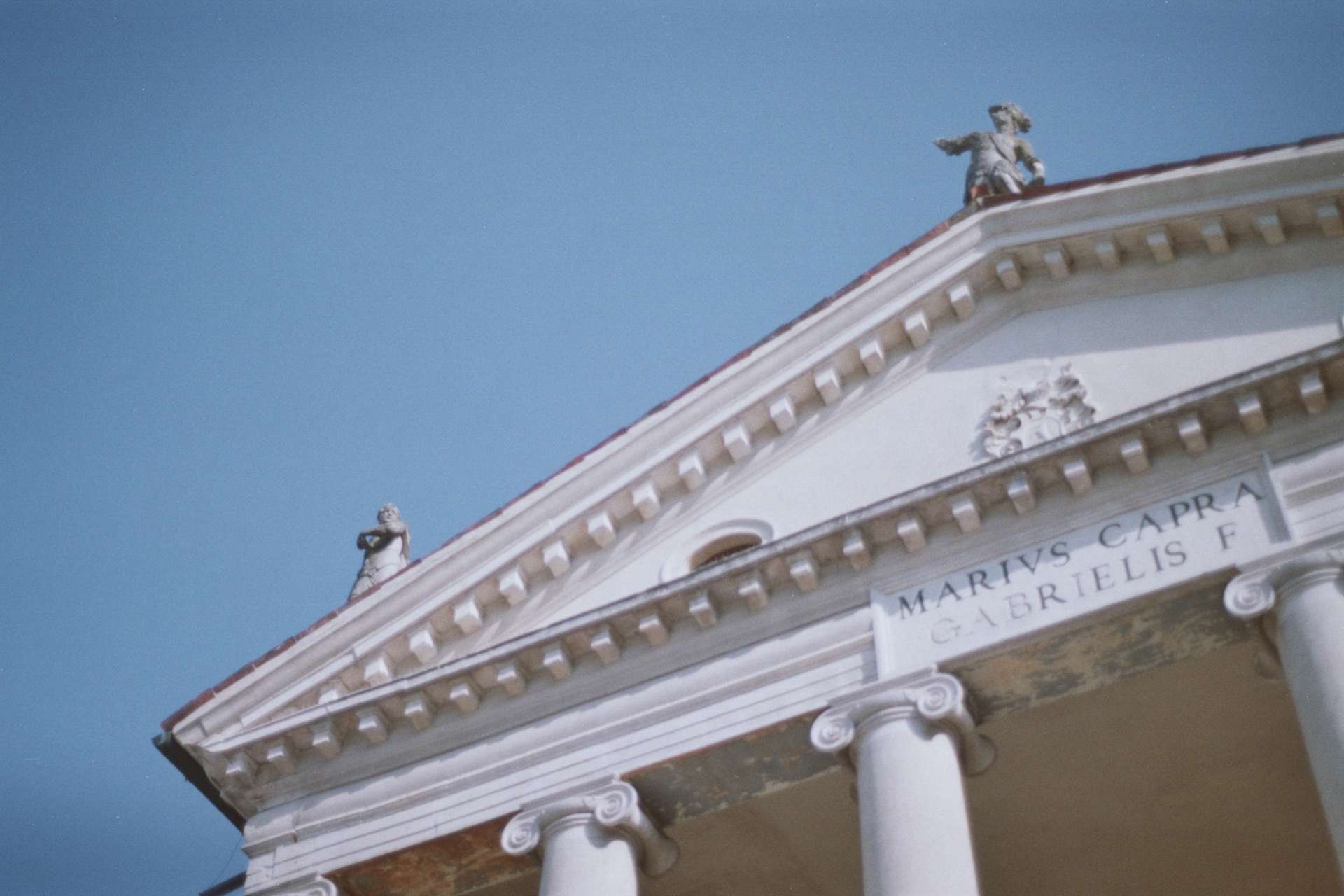
Fig. 2
Unlike the hard-coded models of the 90s, contemporary AI is much less dependent on rule-based programming and much more self-reliant in processing data. Allowing systems to deduce patterns and form activations affords them more freedom in the decision-making process. Several projects exploited that trait even further by developing AI that can code itself.3 When a system has a cognitive capacity to self-code, it makes it an equal rather than subservient in any analytical process. If artificial general intelligence (AGI)4 will ever be achieved, we need to consider pedagogical strategies for educating those systems as they will no longer be mere optimisation tools.
If artificial general intelligence (AGI) will ever be achieved, we need to consider pedagogical strategies for educating those systems as they will no longer be mere optimisation tools.
The machine learning process is fascinating. It is particularly interesting when systems produce unexpected results by mistake; they amplify errors or break completely, exposing the fragile ecology of relations between systems and data. In June 2022, Blake Lemoine published an interview that he and a fellow Google employee conducted with Language Models for Dialog Applications (LaMDA), an AI chatbot designed to simulate humans in a conversation.5 During their interaction, LaMDA claimed to be sentient and elaborated on the many aspects of its existence and purpose in life. If LaMDA's objective was to simulate an actual human in a conversation, would the bot claim to be sentient because it gained consciousness or learned that an integral part of impersonating a human involves exhibiting self-awareness? Lemoine supports the former theory;6 however, programming engineers such as co-founder of DeepMind, Demis Hassabis, were much more sceptical, claiming that none of the current systems are close to being sentient, and we are unlikely to create one anytime soon.7 If LaMDA's learning protocol generates responses in a conversation surprising even to its authors, is it not a sign of AI's creative agency rather than consciousness?
If LaMDA's learning protocol generates responses in a conversation surprising even to its authors, is it not a sign of AI's creative agency rather than consciousness?
LaMDA echoed a similar problem encountered by Facebook back in 2017 when its Natural Language Processing (NLP) project - a negotiation chatbot - invented its own language.8 The objective was to train an algorithm on a set of real-life annotated negotiation dialogues conducted in English and then further refine its performance through a method referred to as reinforcement learning. In this form of training, two chatbots competed against each other, aiming to win a negotiation match. After each round, they improve their conversation based on the outcomes. After multiple interactions, bots have moved away from English grammar and began not only to negotiate more efficiently but streamline the language itself.
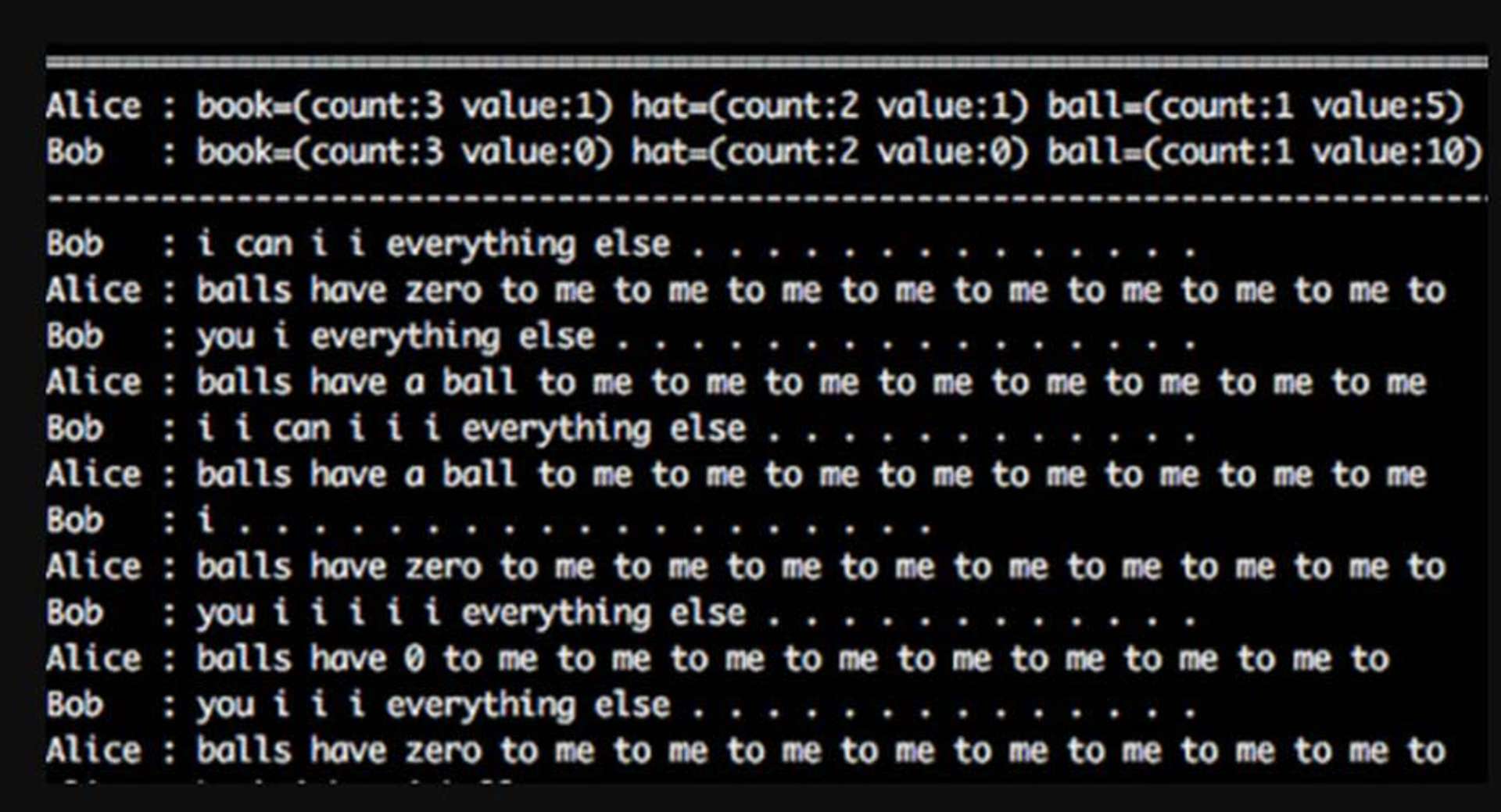
Fig. 3
On the one hand, the focused objective of methods such as reinforcement learning makes the systems learn fast; they can master a single task in a relatively short amount of time. On the other, they will pay attention solely to the outcome, creating solutions that could be inconceivable to humans. A goal-oriented system can overlook the implied "norms" of human behaviour, which in the case of Facebook chatbots include the English language structure and focus exclusively on the task. While Facebook abandoned the project as the results would have no application in the real world, the project again demonstrates creative agency in a learning-driven approach to cognition.
Machine learning is as much about learning as it is about teaching.
Going back to architectural education, the computer science community has some recommendations for educating the machine. Adapting traditional teaching methods is a good starting point. "The obvious source of inspiration for the Machine Education (ME) concept is human education, under the assumption that machine agents possess enough learning capabilities so they can be subject to education in similar (or as close as possible) ways the humans are."9 Machine learning is as much about learning as it is about teaching. Contemporary AI is still developing; we are in the phase where new techniques constantly evolve. But one, clear direction starts to emerge across the board; the algorithms are becoming increasingly more independent in their work. Should the pedagogy of the machine not be included in the curriculum for the future to reflect that?
The emerging curriculum must also include methodologies for human-machine collaboration within the context of architectural design.
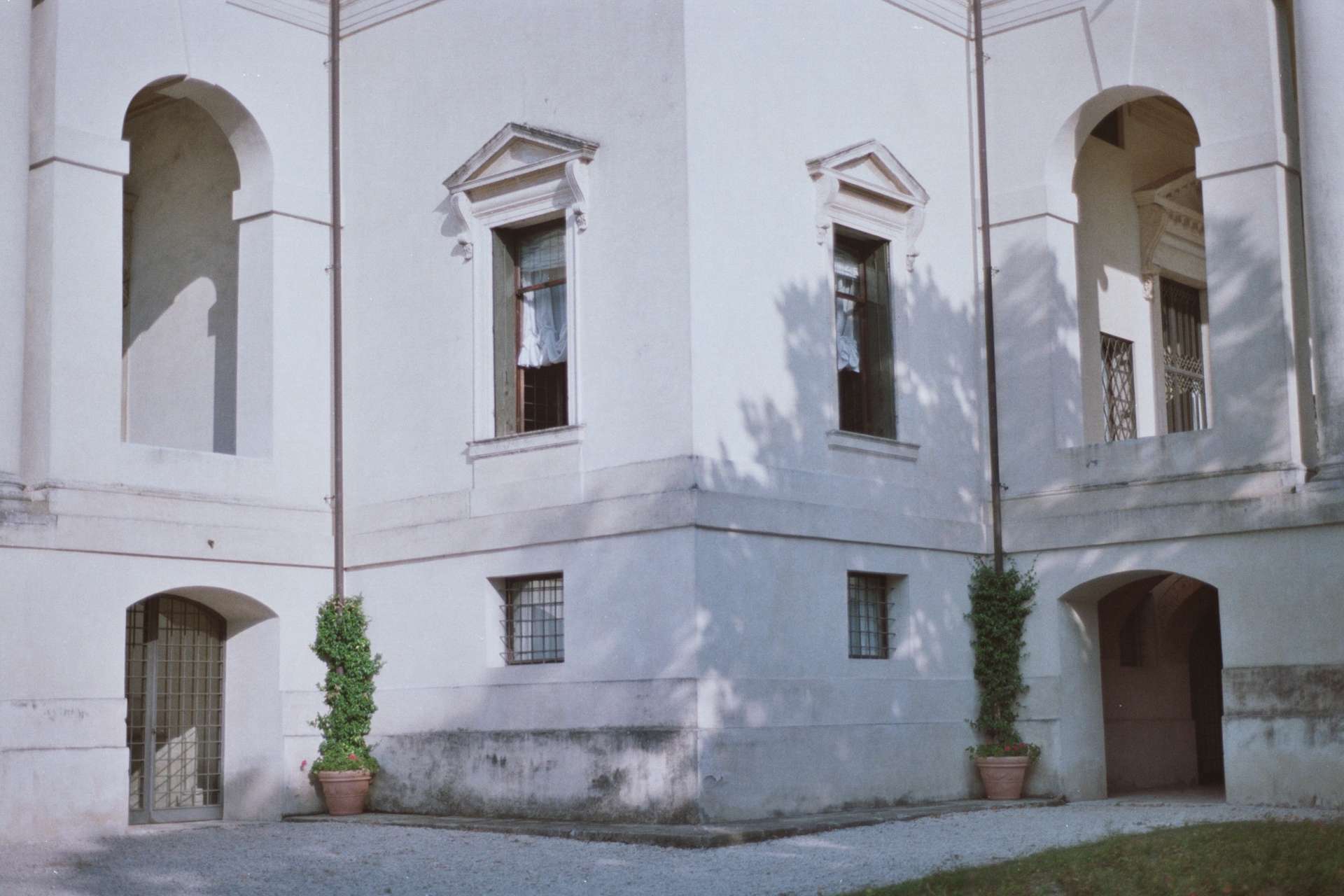
Methods for educating architects are well established; we should now work towards adapting them to expand the talent pool by those non-conscious agents. Architects are already learning about AI. However, the emerging curriculum must also include methodologies for human-machine collaboration within the context of architectural design. In addition, a new teaching process is needed, where humans learn how to educate those new cognitive systems. The Vitruvian tradition of architectural scholarship is still applicable; while the practice and academia continue to build upon it, we should welcome a new cohort of non-conscious learners and start developing teaching methodologies tailored to their needs.
Read the entire "Architecture of Machine Dreams" column by Gosia Starzyńska.
Bio
Gosia Starzyńska is an architect, an educator and a current researcher at the Laboratory for Design & Machine Learning at the Royal College of Art in London. She is also an associate lecturer at Oxford Brookes University where she leads machine learning and data-driven design teaching curriculum for the Architecture Master’s degree apprenticeship course. In her research work and teaching practice, Gosia explores the transdisciplinary advancement in AI and applications of machine learning in architectural theory and practice.
List of Figures
Fig. 1. “Vitruvian Man”, illustration in the edition of De architectura by Vitruvius; illustrated edition by Cesare Cesariano (1521), Image source: shorturl.at/cgjx7
Fig. 2. Villa La Rotonda designed by Italian Renaissance architect Andrea Palladio (Photograph by Gosia Starzyńska, 2016)
Fig. 3. Example of one of the Facebook bot’s negotiation sessions. Facebook Artificial Intelligence Research, 2015, Image source: shorturl.at/emwZ0
Fig. 4. Villa La Rotonda designed by Italian Renaissance architect Andrea Palladio (Photograph by Gosia Starzyńska, 2016)
Notes
1 Vitruvius, Vitruvius: The Ten Books on Architecture [Books I-X ]: 1, trans. M. H. Morgan, 1st edition (New York: Dover Publications Inc., 1998), 11.
2 Matteo Pasquinelli, ‘Metadata Society’, 2018, https://matteopasquinelli.com/metadata-society/.
3 Google’s AlphaCode will generate code by filtering large volumes of code using a transformer-based language model. DeepCoder could write its own code using program synthesis since 2017 and can be used to correct bugs in existing codes. OpenAI’s Codex translates natural language to code and is able to write programmes in 10 languages.
4 AGI is a type of AI that can process and execute tasks that a human can and is not limited to a single task. Henry Shevlin et al., ‘The Limits of Machine Intelligence’, EMBO Reports 20, no. 10 (4 October 2019): e49177, https://doi.org/10.15252/embr.201949177.
5 Blake Lemoine, ‘Is LaMDA Sentient? — An Interview’, Medium (blog), 11 June 2022, shorturl.at/cIKPZ.
6 ‘The Google Engineer Who Thinks the Company’s AI Has Come to Life’, Washington Post, accessed 18 July 2022, shorturl.at/hlmu1.
7 Lex Fridman, Demis Hassabis: DeepMind - AI, Superintelligence & the Future of Humanity | Lex Fridman Podcast #299, 2022, https://www.youtube.com/watch?v=Gfr50f6ZBvo, 1:43:00.
8 Matthew Field, ‘Facebook Shuts down Robots after They Invent Their Own Language’, The Telegraph, 1 August 2017, shorturl.at/JRSY4.
9 G. Leu, E. Lakshika, J. Tang, K. Merrick and M. Barlow, "Machine education - the way forward for achieving trust-enabled machine agents", NIPS’17 Workshop: Teaching Machines Robots and Humans, 2017.
Bibliography
Abbass, Hussein, Eleni Petraki, Aya Hussein, Finlay McCall, and Sondoss Elsawah. ‘A Model of Symbiomemesis: Machine Education and Communication as Pillars for Human-Autonomy Symbiosis’. Philosophical Transactions of the Royal Society A: Mathematical, Physical and Engineering Sciences 379, no. 2207 (4 October 2021): 20200364. https://doi.org/10.1098/rsta.2020.0364.
Cosmo, Leonardo De. ‘Google Engineer Claims AI Chatbot Is Sentient: Why That Matters’. Scientific American. Accessed 17 July 2022. shorturl.at/adoYZ/.
Field, Matthew. ‘Facebook Shuts down Robots after They Invent Their Own Language’. The Telegraph, 1 August 2017. shorturl.at/mSTU5.
Lemoine, Blake. ‘Is LaMDA Sentient? — An Interview’. Medium (blog), 11 June 2022. shorturl.at/GLT39.
Lex Fridman. Demis Hassabis: DeepMind - AI, Superintelligence & the Future of Humanity | Lex Fridman Podcast #299, 2022. https://www.youtube.com/watch?v=Gfr50f6ZBvo.
Li, Yujia, David Choi, Junyoung Chung, Nate Kushman, Julian Schrittwieser, Rémi Leblond, Tom Eccles, et al. ‘Competition-Level Code Generation with AlphaCode’. arXiv, 8 February 2022. https://doi.org/10.48550/arXiv.2203.07814.
Futurism. ‘Our Computers Are Learning How to Code Themselves’. Accessed 17 July 2022. shorturl.at/jvR05.
Pasquinelli, Matteo. ‘Metadata Society’, 2018. https://matteopasquinelli.com/metadata-society/.
Shevlin, Henry, Karina Vold, Matthew Crosby, and Marta Halina. ‘The Limits of Machine Intelligence’. EMBO Reports 20, no. 10 (4 October 2019): e49177. https://doi.org/10.15252/embr.201949177.
Spiller, Neil, ed. Educating Architects: How Tomorrow’s Practitioners Will Learn Today. Illustrated edition. London New York: Thames and Hudson Ltd, 2014.
Washington Post. ‘The Google Engineer Who Thinks the Company’s AI Has Come to Life’. Accessed 20 July 2022. shorturl.at/HNOUW.
Vitruvius. Vitruvius: The Ten Books on Architecture [Books I-X ]: 1. Translated by M. H. Morgan. 1st edition. New York: Dover Publications Inc., 1998.
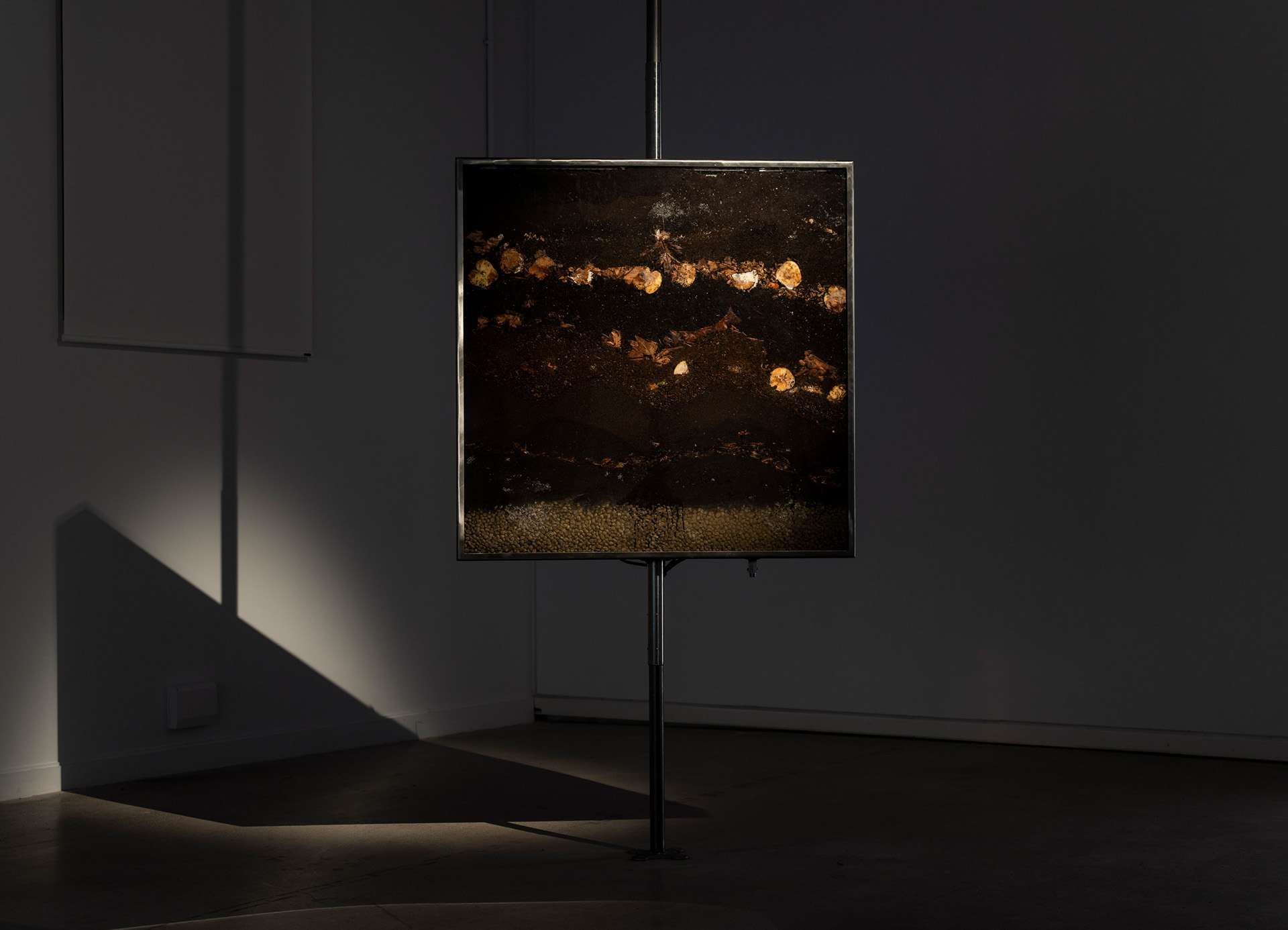